RISE with SAP
Syntax Compass for SAP
The path to RISE with SAP can be complex. Syntax Compass will guide you toward an efficient and successful strategy.
Who we are
Syntax is a global provider of IT services and cloud services. We mainly support medium-sized enterprises in industrial sectors to master a diverse range of IT challenges.
Alongside cloud computing, application management services, digital manufacturing and the modern workplace, Syntax focuses on comprehensive services relating to the SAP systems. From initial consultation and planning, via implementation to the operation of regional and globally distributed hybrid SAP landscapes, Syntax offers a full range of services. At Syntax we plot out our customers’ routes to S/4HANA, helping them to achieve their digital transformation through SAP within the Industrial IoT. We also consult on the operation of SAP in the cloud, be it private, public or hybrid.
400+
Customers
100+
Worldwide Installations
20+
Sectors
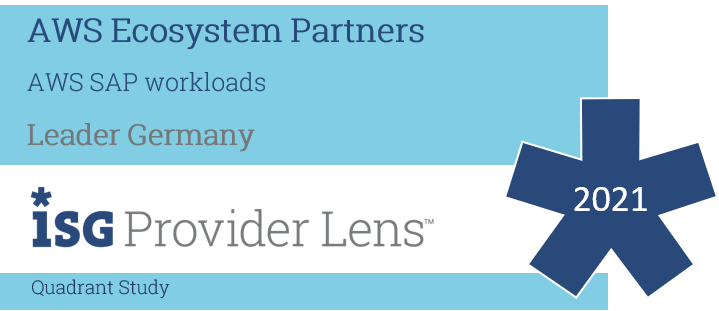
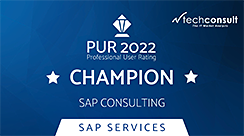
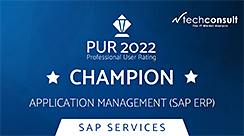
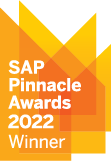
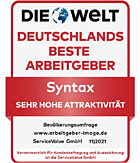